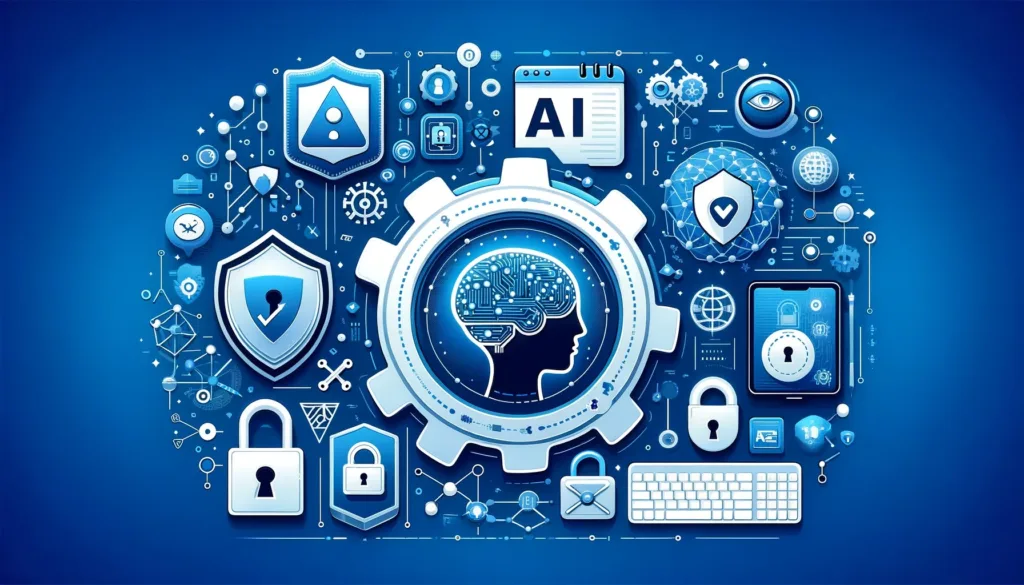
Table Of Contents
- Introduction
- The Importance of AI TRiSM
- Key Components of AI TRiSM
- Best Practices for Implementing AI TRiSM
- Case Studies and Real-World Examples
- Conclusion
- References
Introduction
Artificial Intelligence (AI) is revolutionizing industries, but its rapid growth brings new challenges. Ensuring AI Trust, Risk, and Security Management (AI TRiSM) is critical for mitigating risks and maintaining public confidence. This article explores the importance of AI TRiSM and provides best practices for its implementation.
The Importance of AI TRiSM
AI systems are becoming integral to decision-making processes across various sectors. However, they can be unpredictable and opaque, leading to potential risks. Ensuring trust and security in AI systems is essential for their reliable and ethical use. Unmanaged risks can lead to severe consequences, including data breaches, biased outcomes, and loss of user trust. Therefore, businesses must prioritize AI TRiSM to safeguard their operations and maintain stakeholder confidence.
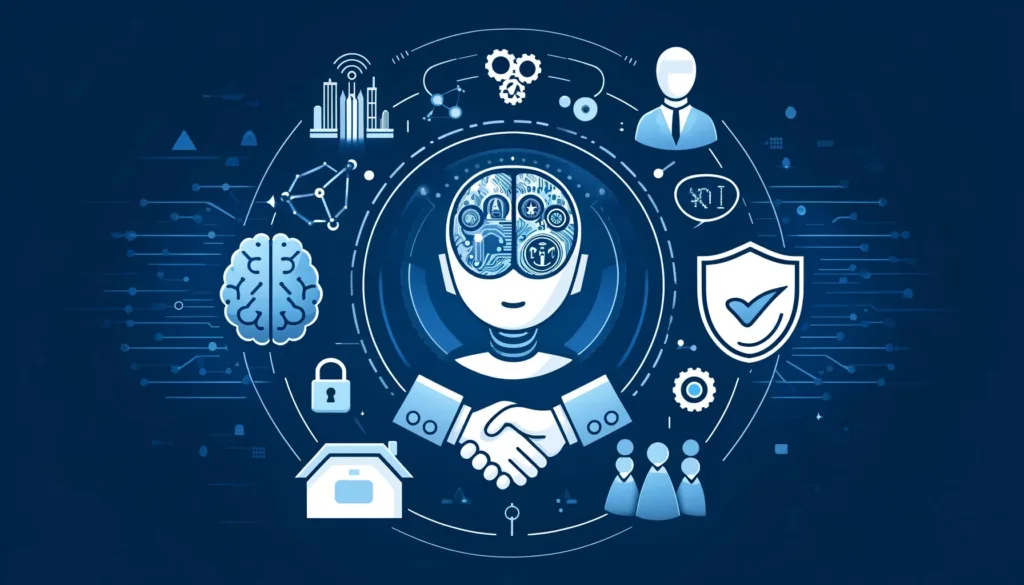
Key Components of AI TRiSM
- Trust Management
- Transparency: Ensuring AI decisions are explainable and understandable to users.
- Reliability: Building user confidence through consistent and dependable AI behavior.
- Ethical AI: Implementing ethical guidelines to ensure AI systems adhere to societal values.
- Risk Management
- Risk Identification: Identifying potential risks associated with AI deployment, including data privacy concerns and algorithmic bias.
- Mitigation Strategies: Developing strategies to manage and mitigate identified risks effectively.
- Compliance: Ensuring AI systems comply with relevant regulations and standards.
- Security Management
- Cybersecurity Measures: Protecting AI systems from cyber threats through robust security protocols.
- Data Protection: Ensuring the integrity and confidentiality of data used by AI systems.
- Incident Response: Establishing procedures for responding to security breaches and incidents.
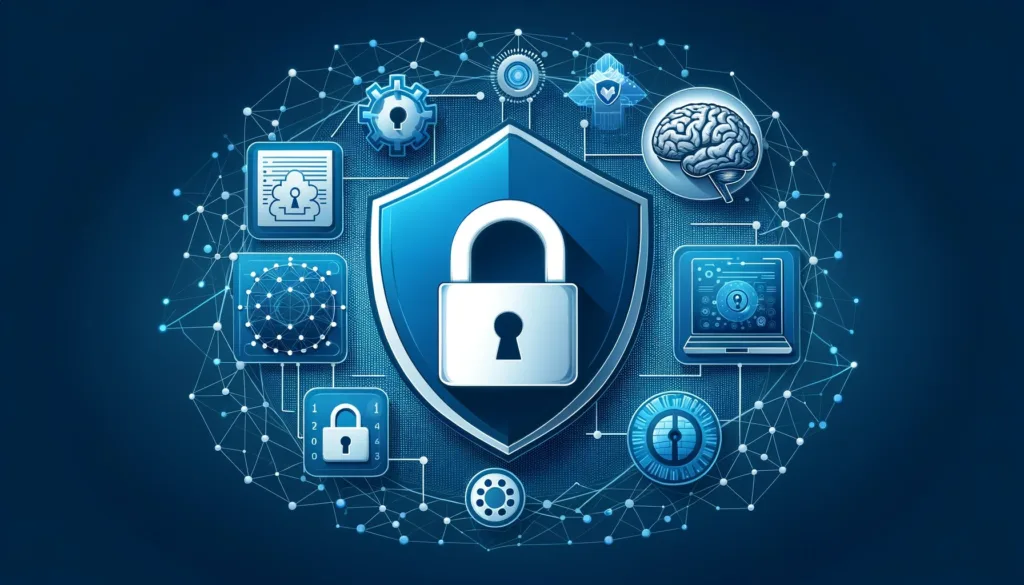
Best Practices for Implementing AI TRiSM
- Adopt Explainable AI (XAI)
- Why it Matters: Explainable AI enhances transparency by making AI decisions understandable. It helps build user trust and accountability.
- How to Implement: Use techniques such as model interpretability and post-hoc explanations to clarify AI decision-making processes.
- Conduct Regular Audits
- Why it Matters: Regular audits ensure compliance with regulations and identify potential vulnerabilities.
- How to Implement: Establish a routine audit schedule and involve third-party auditors for unbiased assessments.
- Enhance Cybersecurity Measures
- Why it Matters: Robust cybersecurity measures protect AI systems from cyber attacks, ensuring data integrity and confidentiality.
- How to Implement: Use encryption, secure protocols, and continuous monitoring to safeguard AI systems.
- Develop a Comprehensive Risk Mitigation Plan
- Why it Matters: A thorough risk mitigation plan addresses potential threats and outlines strategies to manage them effectively.
- How to Implement: Identify risks, assess their impact, and develop mitigation strategies. Regularly update the plan based on new insights and developments.
- Engage Stakeholders in AI Governance
- Why it Matters: Involving stakeholders ensures that AI systems align with organizational goals and societal values.
- How to Implement: Create AI governance committees that include diverse stakeholders, including technologists, ethicists, and end-users.
- Foster an Ethical AI Culture
- Why it Matters: Promoting an ethical AI culture ensures that AI systems are developed and used responsibly.
- How to Implement: Provide training on ethical AI principles and integrate ethics into the AI development lifecycle.
- Implement Continuous Monitoring and Evaluation
- Why it Matters: Continuous monitoring helps detect and address issues promptly, ensuring the ongoing reliability and security of AI systems.
- How to Implement: Use automated monitoring tools and establish a feedback loop for continuous improvement.
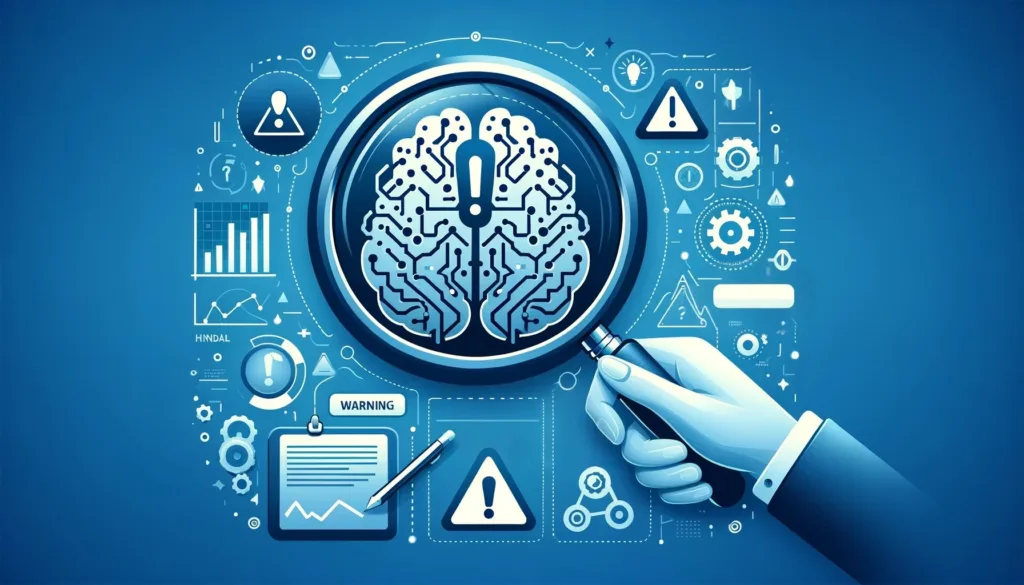
Case Studies and Real-World Examples
- Healthcare: AI systems in healthcare must ensure patient data privacy and unbiased medical recommendations. Regular audits and ethical guidelines are crucial.
- Finance: Financial institutions use AI for fraud detection and credit scoring. Ensuring transparency and security is vital to maintain customer trust.
- Retail: AI in retail personalizes shopping experiences. Protecting customer data and ensuring fair recommendations are key priorities.
Conclusion
AI TRiSM is essential for the sustainable and ethical growth of AI technologies. By implementing trust, risk, and security measures, businesses can harness the full potential of AI while safeguarding their operations and maintaining public confidence.
References
- Gartner Top 10 Strategic Technology Trends 2024 (Gartner)
- Top 10: Technology trends for 2024 (Home of Technology News)
- 22 New Technology Trends for 2024 (Simplilearn.com)
Implementing AI TRiSM ensures that AI advancements are secure, trustworthy, and beneficial for all.
My admiration for your creations is as substantial as your own sentiment. The visual presentation is tasteful, and the written content is sophisticated. Yet, you seem uneasy about the possibility of presenting something that may cause unease. I’m confident you’ll be able to resolve this issue efficiently.